Transcribing Flemish
Let our tool write your meeting notes for you. Us humans aren't very good at multitasking, especially when it comes to capturing concise, straightforward notes during a meeting. Imagine trying to keep up with the fast-paced conversation while ensuring every critical point is documented accurately. It's a skill few possess, and it's even more challenging if the conversation is happening in West-Flemish. Existing transcription models like Whisper, AssemblyAI, and Google Cloud Speech-To-Text have made significant strides in general speech-to-text technology. However, they fall short when it comes to Flemish (Vlaams), especially its distinct dialect, West-Flemish (West-Vlaams). Our tool aims to bridge this gap.
We compiled a comprehensive validation set consisting of 19,252 recordings. These were sourced from well-known datasets like Mozilla's Common Voice, Google's Fleurs, Facebook's VoxPopuli, and our own bespoke recordings in Flemish and it's dialects (West-Flemish, Antwerps, ...).
Leading up to a validation set of 19.252 recordings.
We calculated the performance a couple of models using two metrics:
WER (Word Error Rate): This is a common metric used to evaluate the accuracy of speech recognition systems. It measures the percentage of words that were incorrectly transcribed by comparing the transcribed text to the reference text. A lower WER indicates better performance, as it means fewer transcription errors.
CER (Character Error Rate): Similar to WER, the Character Error Rate measures the accuracy of a speech recognition system at the character level. It calculates the percentage of characters that were incorrectly transcribed, again by comparing the transcribed text to the reference text. This metric is especially useful for languages where character-level precision is crucial, such as languages with complex scripts. A bit less important than CER (especially in Dutch), because the meaning of the word will probably remain in tact.
We decided to run our benchmarks against these publicly available models, next to our own model:
Comparison | whisper-large-v3 | seamless-m4t-v2-large | intellua-large-vlaams |
Word Error Rate | 20,31 | 27,77 | 13,77 |
Character Error Rate | 13,96 | 15,04 | 7,13 |
Load time (seconds) | 22 | 12,19 | 7 |
Eval time (seconds) | 2022 | 896 | 812 |
Memory Req. | ~10GB RAM | ~5GB RAM | ~3GB RAM |
Our model scores better on flemish, is faster and can even run locally on an iPad or iPhone.
Interested in trying the model yourself, or integrating it in your own product? Get in touch!
Want a solution like this?
Enter your email here and we'll get in touch with you.
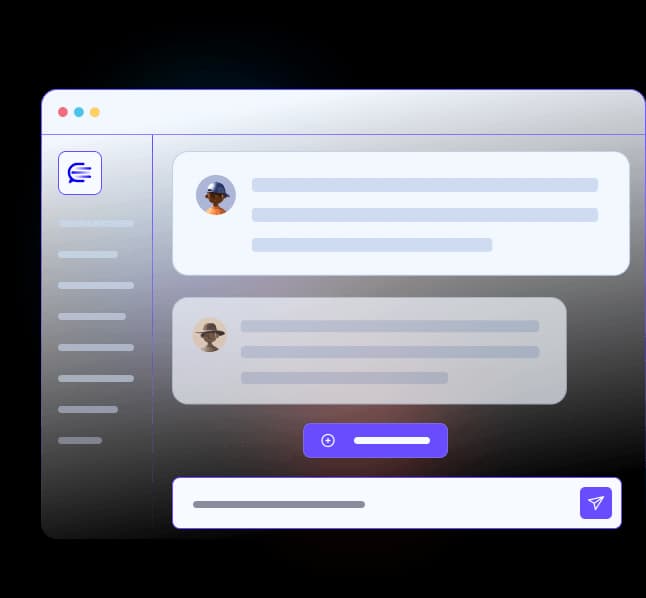